More and more decisions in companies are being made on the basis of data. Data literacy is therefore becoming a crucial skill. In order to train employees in data literacy, companies are increasingly investing in data literacy concepts. In this interview, Christian Schneider, Head of Data & Analytics at QuinScape GmbH, discusses how data literacy fits into a successful data strategy and sheds light on recipes for success as well as the pitfalls of becoming data-driven as a company.
Data literacy in companies: Successes and stumbling blocks
In your presentation at this year's TDWI, you talk very provocatively about bullshit and bias in data literacy initiatives. What are you alluding to?
Bringing data literacy or data competence into companies is currently very much in vogue. Many companies that are moving in the direction of "data driven" are trying to develop data strategies on their own, which also includes creating data literacy.
However, building data literacy among employees usually goes hand in hand with a cultural change in the company. It is therefore more helpful to do this with experienced support. What we observe in our projects is that for many companies, "data-driven" means teaching all employees how to work with data and making as much data as possible available. This often leads to the point where companies overshoot the mark and things start to go wrong.
Data democratization vs. targeted data provision: smart use of 'data driven'
What exactly is the problem here? What expectations do companies have of such initiatives and what do they expect from them?
Initiatives to become data-driven involve a significant investment for companies. Understandably, such an investment should then also pay off. It's like a new car: you drive a lot to get the feeling that the car is of real use. But being a data-driven company does not mean giving all employees as much company data as possible. That is often a misunderstanding of data democratization.
When I am confronted with too much data as an employee, but don't actually know what is relevant for me, I often fall victim to "FOMO", the phenomenon of "fear of missing out". This means that I waste a lot of time looking at all kinds of data in the hope of gaining as many insights as possible as quickly as possible.
However, being data-driven means preparing and making data available for specific processes. In order to make better decisions based on this data, it is important to train employees to be able to handle data critically and competently.
This is precisely where successful data literacy initiatives come in: enabling people to deal critically with data in order to ideally make better decisions.
What exactly is the danger of being confronted with too much data?
This is where the psychological effect of the "tyranny of small decisions" often comes into play. This is the case when you get too much detailed data too quickly and start making small adjustments to decisions at a strategic level. The more small decisions you have, the more often you tend to change course just a little at a time. However, if you frequently make minor adjustments to the course, you may end up veering off course.
In other words, presenting too much data in the hope of having a complete view of the database is usually counterproductive. It is better to distil the data down to the necessary level. Only when further data is required for a decision is it useful to look into the big box. But not before, because it may tempt you to make small decisions that lead away from the actual goal.
Data governance as a guard rail for data-based decisions
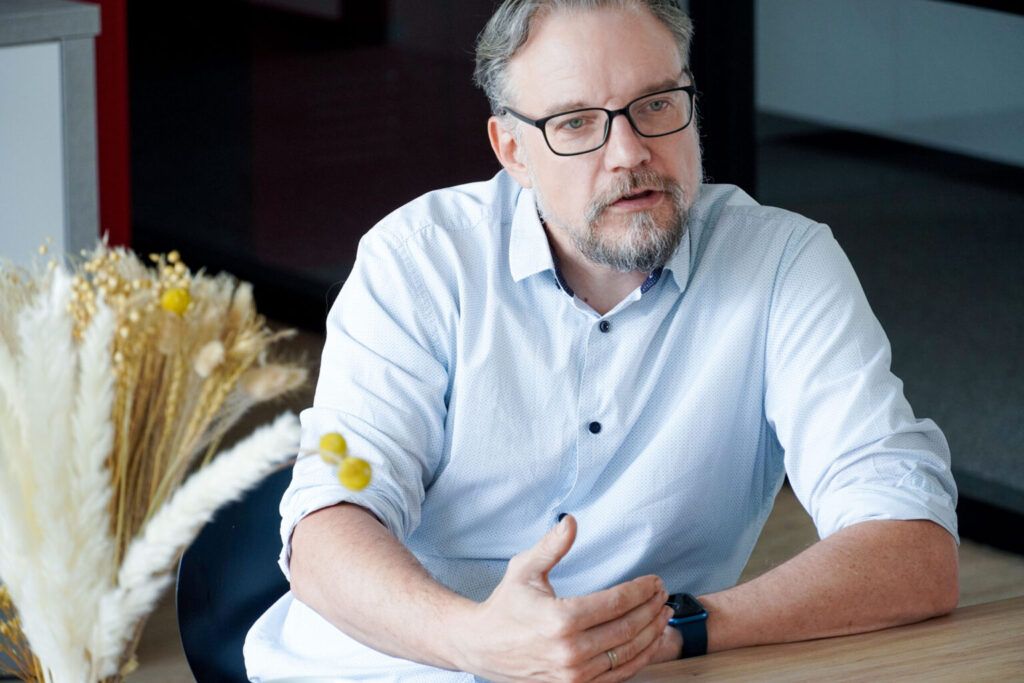
So should data literacy start with being able to critically decide which data is important for my decisions?
Exactly. That brings us to the area of data governance. Here I find the image of data governance as guard rails very appropriate. Guard rails do not mean a restriction, they are rather there to avoid a disaster and not to get off the road.
Data governance has this function within a data strategy. Every area of a company needs its data. Defining this is often already regulated in many companies. What often does not yet work is making data available across departments where it is needed.
Ultimately, it's about making well-founded decisions based on data that I can trust and making the actual decision-making process more efficient. In projects, we often see concerns about additional work in addition to day-to-day work, as employees have to learn how to use new tools or data in general.
In our view, inefficiency only occurs when data is provided without an accompanying concept, which can have psychological effects such as overburdening or even a loss of power among employees.
You have to keep reminding yourself that this is a cultural change that needs to be handled responsibly. A data strategy as a roadmap with a clear goal, data governance as a guardrail to ensure you don't stray from the path and data literacy as a driver's license to make data-based decisions with as little risk of accidents as possible all help here.
The four phases of data-driven transformation
What does an optimal start look like for you to become data driven as a company?
Companies invest a lot of money in data every day, in collecting, storing and maintaining it. For a long time, the principle was to simply store everything possible in terms of data. After all, storage space costs nothing. The unused data is known in the industry as "dark data". Its share is not insignificant. The way in which companies deal with data as an economic asset must change fundamentally.
We divide the process into four phases. The first phase is an inventory. Here we see different levels of maturity in companies: from those that have not yet dealt with it at all to those that have already identified their data silos and beyond.
The second phase comprises the definition of a data strategy. This also includes the gradual development of data governance and a data literacy concept. The third phase is implementation, both technical and organizational. Finally, the fourth phase, often forgotten: the operational phase, i.e. maintaining the quality achieved in the first three phases through a continuous process.
This is where data literacy is a decisive factor in being able to scrutinize data quality. This does not have to be demanded of all employees in equal measure. Companies can set up targeted data communities to take on this role.
The lighthouse approach: persuasion through concrete results
Do you have a practical example of how something like this was handled well?
What works very well is to start with a lighthouse project. A project like this has the effect of convincing people in the company because concrete positive effects are clearly visible. In one case, we shared data from clinical research so that the data quality increased through automation and the added value from the previously non-integrated data led to more and more departments wanting to participate.
Involving people from the outset is the decisive factor when it comes to cultural change anyway. What we therefore always aim for in our projects is the intrinsic motivation of employees to participate. Whether this works depends very much on the will and culture of each company.
Initially, there is almost always a certain degree of rejection, because change often arises out of necessity and this necessity is not immediately apparent to everyone. As in the example above, someone has to have the courage to take the first step. However, when I as an employee realize that the change has a positive impact on me, my interest often turns into a desire to actively participate in the change.
That is the effect we achieve with the lighthouses. Transparent and open communication is of course essential.
Data literacy as the key to a data-driven future
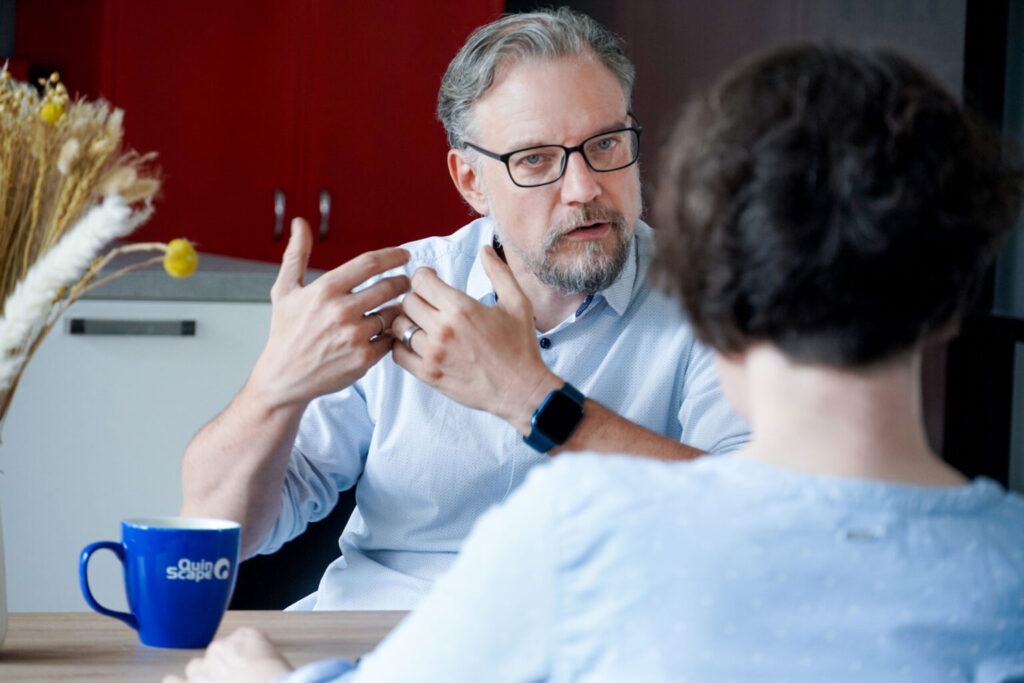
What role does technology play here?
The choice of technology depends heavily on the type of company. A manufacturing company needs different tools than a consulting firm. Technologies are tools for us to achieve the goal of becoming data driven. On the other hand, it doesn't work to say: I use this tool, so I am data driven.
When is a company data driven?
We approach consulting with a simple and striking metric that gives rise to many questions: A company is data-driven when the data in the company no longer needs to be questioned in every meeting before decisions are made.
Of course, a critical approach to data and questioning its significance is still important. However, this is about data quality that no longer needs to be questioned, but enables people to make reliable decisions. Being data-driven means using the available data quickly and securely.