This year, our NEXT STEP FORWARD online event will focus on key practical tips and recommendations for the first 100 days of your first or next machine learning project.
Learn from our top speakers how you can organize your entry into the topic of machine learning. Benefit from our blueprints, which will provide you with targeted support during conceptualization. Use the experience report to avoid obstacles at an early stage in the future and gain lasting inspiration. We show you which requirements your data management should fulfill and present tried-and-tested architectures. How all this can also work in an AWS cloud rounds off our exciting 90-minute offer for you.
Agenda
The ubiquity of machine learning: the question is not if but when
Speaker from QuinScape GmbH: Dr. Gero Presser
Machine learning is becoming more and more mainstream today. Until a few years ago, self-learning programs were mainly a topic at universities. Today, machine learning is playing an increasingly important role in products and services.
Business life is increasingly influenced and driven by machine learning. The possibilities and capabilities are diverse and flexible.
Self-learning machines can thus recognize damage or error patterns in production faster than humans. The ability to process complex correlations between the input and output of large volumes of data is just one of the major advantages of machine learning.
Therefore, the question is not whether machine learning will reach your industry, but when you can best recognize the benefits for your company and make use of the many possibilities.
The first 100 days of data science and beyond: How to get started with machine learning
Speaker from ixto: Dr. Simon Birkholz
Due to their technical complexity and often only roughly predictable prospects of success, data science projects present an unmanageable planning challenge. In addition to questions about data handling and model development, an overall concept for a practicable project must be created. For this long-term success, the first steps in particular are of critical importance. Thanks to increasing experience, however, ML and AI projects are becoming more and more reliable.
In this article, we will guide you through the blueprint for your next data science project. We look forward to discussing tried-and-tested concepts and sharing your experiences with you.
How we successfully introduced machine learning: A field report with practical tips for design
Speaker from ixto: Franziska Rupprecht
The first data science project is a particular challenge for companies of all sizes. Experts from a wide range of areas, from corporate IT to the specialist department, often have to be involved. Due to this particular complexity, proven methods from software development are often not sufficient to conceptualize a data science project.
In this article, we describe the conception of a machine learning project from the formulation of the task, through agile team organization, to integration into the finished application. Learn from examples of obstacles that have been overcome and find inspiration from tried-and-tested solutions.
Why data management and data quality are crucial for successful machine learning
Speaker from QuinScape: Phil Moston
Data management and, in particular, reliable data are the basic prerequisites for machine learning. In this presentation, we will explain the pitfalls involved, which architectures and procedures are currently best practice and how the classic business intelligence world can be successfully combined with the field of data science.
How technology becomes a minor matter with the right tools AWS as a cloud data platform for machine learning
Speaker from AWS: Christian Elsenhuber
Using machine learning in productive operations sounds great, but many companies don't get beyond experimentation. In this presentation, we will shed light on how to achieve results quickly and cost-effectively with the wide range of services that AWS provides in the field of machine learning and data management in general.
Lessons learned: Practical tips and recommendations for successfully getting started with machine learning
Speakers from QuinScape GmbH: Christian Schneider, Dr. Gero Presser
We recap the event, summarize the most important findings and provide an outlook for the next steps.
Speakers
Dr. Simon Birkholz
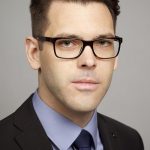
Dr. Simon Birkholz is Managing Director of ixto GmbH. As a consultant and team leader, he has primarily advised corporate clients in the energy and automotive sectors on data analysis projects in the cloud. His current focus is on the realization of data science projects.
Franziska Rupprecht
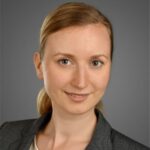
Franziska Rupprecht studied environmental sciences and works as a data scientist at ixto GmbH. She primarily implements machine learning and AI projects in the energy sector.
Christian Elsenhuber
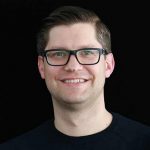
Christian Elsenhuber has been working in technology consulting for more than 15 years as a requirements engineer, project manager and IT architect. He has experience from projects in batch processing, web development and managed services. As a Partner Solutions Architect at AWS, his role is to advise AWS service partners on new technologies and architectures and to support them in developing their next-generation AWS-based service offerings.
Philip Moston
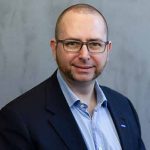
Philip Moston is Principal Consultant for Data & Analytics at QuinScape GmbH and is responsible for the design of customized data architectures. For more than three years, Philip has also been working intensively on the aspect of "data virtualization" and for 20 years he has been helping QuinScape's customers to gain insights from data.
To the recording of the event: https://sites.quinscape.de/next-step-forward-erfolgreicher-einstieg-in-machine-learning